What to know
Your EHDI Information System (EHDI-IS) is a powerful tool that can directly help ensure that all children receive recommended and time-sensitive EHDI services. Inaccurate data in an EHDI-IS can impede the delivery of services by preventing you from realizing what follow-up is needed. Equally important as having complete and high quality data is using the information to support tracking and surveillance. An EHDI-IS with accurate data helps you know which infants have been screened and which ones need follow-up services. This information helps you better determine where to focus your follow-up efforts and supports the provision of services to infants in need.
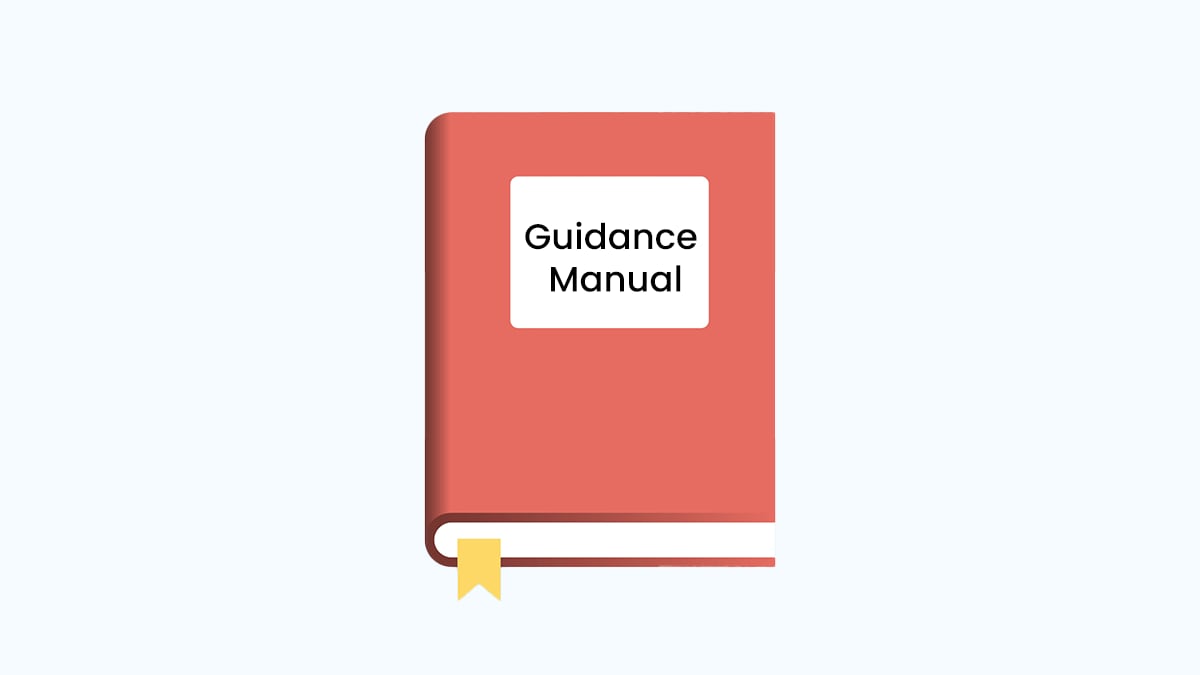
Chapter objectives
This chapter will help you to
- Use data to support and confirm the delivery of EHDI services, to present program status/ performance to stakeholders, and to inform providers about their performance;
- Understand the levels of analysis (e.g., data analysis plan, analysis file, available statistical software) and how to use these to report findings;
- Understand the possible questions EHDI data can answer;
- Understand the need for standardizing data reporting; and
- Understand the importance of an audience for data dissemination.
Overview
Your EHDI Information System (EHDI-IS) is a powerful tool that can directly help ensure that all children receive recommended and time-sensitive EHDI services. As discussed in Chapter 1, jurisdictions can use the EHDI Functional Standards 1, which are detailed on the CDC website, as a framework for determining the operational, programmatic, and technical functional requirements for a complete EHDI-IS. Maximizing the benefits of an EHDI-IS, the EHDI program can ask the following key questions;
- Does the EHDI-IS capture complete and high-quality data?
- Is your program able to use the data your EHDI-IS captures?
Inaccurate data in an EHDI-IS can impede the delivery of services by preventing you from realizing what follow-up is needed. To consistently obtain accurate data, it is important that you have clear reporting guidelines, data definitions, and support from the stakeholders that have the data of interest (e.g., birth hospitals, audiologists, physicians, and early intervention [EI] programs).
Equally important as having complete and high quality data is using the information to support tracking and surveillance. An EHDI-IS with accurate data helps you know which infants have been screened and which ones need follow-up services. This information helps you better determine where to focus your follow-up efforts and supports the provision of services to infants in need. When infants do not receive recommended follow-up, the benefits of newborn hearing screening are diminished.
Using these same data also makes it possible for you to
- Monitor progress;
- Evaluate effectiveness;
- Highlight successes to decision makers;
- Engage stakeholders; and
- Identify areas for improvement.
Using an EHDI-IS only to store data or generate an occasional report does not help lead to the early diagnosis of deaf and hard of hearing children or support a program's work.
Using your EHDI data to ensure services
The early identification and provision of EI for all deaf and hard of hearing (D/HH) infants is the goal of an EHDI program. Accomplishing this involves first screening all infants, followed by diagnostic testing for those not passing the screen, and referral to EI for those identified as being D/HH. The national objectives call for a diagnosis before 3 months of age and starting intervention before 6 months of age. These diagnostic and intervention services are usually delivered in different locations by different providers.
Active use of EHDI program data can ensure that infants receive all the recommended services that make early identification and enrollment in EI possible. You could start by using data to identify any infants shown to be not screened and those that did not pass.
- Following up with the birthing facility when an infant appears not to have been screened can help distinguish between a missed hearing screen and missing documentation.
- Data indicating that infants from a particular facility or region are often not screened may help your program identify potential quality of care or data reporting issues that can then be addressed with those facilities.
- Data indicating that infants from a particular facility or region are often not screened may help your program identify potential quality of care or data reporting issues that can then be addressed with those facilities.
- When the data indicate that an infant did not pass the screening, you can work to ensure rescreening and/or that diagnostic tests are provided, and the results reported. Depending on your program and the applicable rules, this may involve contacting the birth facility, primary care provider of record, audiologist (if known), and/or the infant’s family.
- Routine analysis of data about the documented receipt of rescreening and diagnostic testing may help you determine to what extent and why D/HH infants may not be receiving recommended follow-up services. That information in turn makes it possible to develop solutions to address identified issue(s). A detailed discussion of evaluation and monitoring is provided in Chapter 6.
- Routine analysis of data about the documented receipt of rescreening and diagnostic testing may help you determine to what extent and why D/HH infants may not be receiving recommended follow-up services. That information in turn makes it possible to develop solutions to address identified issue(s). A detailed discussion of evaluation and monitoring is provided in Chapter 6.
- Your program can use the data on infants identified with a permanent hearing loss to help confirm that they are referred and, if eligible, enrolled in EI services. Depending on your program and applicable rules, this may involve checking with the audiologist to see if referral to EI services has been made, providing the name of the infant to the jurisdictional Part C program, and/or contacting the family.
Capturing race and ethnicity in your EHDI data
Any data standards published by the Department of Health and Human Services are required to comply with the Office of Management and Budget (OMB) standards.2 In this document, OMB has documented a set of standardized questions on race and ethnicity as required reporting by federal agencies and recipients of federal funds. The OMB racial categories include American Indian or Alaska Native, Asian, Black or African American, Native Hawaiian or Other Pacific Islander, and White. Individuals may select from one or more racial categories. The OMB categories for ethnicity are Hispanic or Latino, or not Hispanic or Latino.
To ensure data quality, OMB advises that race and ethnicity be collected as two questions, with ethnicity being collected first. These categories represent the minimum standard. It is also encouraged to collect more detailed data on race/ethnicity using the OMB racial categories so that they can be aggregated back to the minimum categories. HHS has developed data standards3 that provide additional subcategories within the OMB standard categories of Asian and Native Hawaiian or Other Pacific Islander, as well as for respondents who are of Hispanic, Latino/a, or Spanish origin.
In addition to race/ethnicity, information on primary language or English language proficiency may be collected. This may indicate a barrier for parents whose babies may not receive appropriate services.
Summarizing EHDI performance
Each year, jurisdictions are asked to voluntarily submit aggregate EHDI data to CDC to demonstrate the jurisdiction’s ability to document and provide EHDI services. However, this is not intended to be the only purpose of the EHDI-IS. The EHDI program could also use the EHDI-IS to collect complete, accurate, and valid follow-up screening, diagnostic and intervention data to aid in its work.
Analysis plans
Before analyzing the data captured by the EHDI-IS to help provide and confirm the delivery of services, and to assess performance, your program would be well advised to develop an overall plan for the types of analyses that will likely be conducted as well as data analysis plans (DAPs)4 for more in-depth analyses.
Programs are advised to develop an overall plan for analyses if one is not in place. If a plan does exist and is missing key components, review and update is recommended. Before developing or updating the plan, the EHDI program in consultation with interested stakeholders can determine priorities for use of the data (e.g., hospital quality assurance, identification of data gaps, demographic-related disparities, policy development, legislative action, program monitoring and evaluation, etc.). Referring to past findings and identifying gaps or areas needing improvement may help guide the plan's direction. Obtaining input from the EHDI Advisory Board will be helpful as the priorities are being developed.
In preparing the overall plan for data analyses, consultation with appropriate persons in the jurisdiction on other items to consider, and/or whether required procedures are already in place is recommended. If there are no procedures in place, you may want to consider developing them. Having guidelines in place related to releasing data is important. For example, guidelines could address how to respond to requests for data from external sources such as university researchers. Examples of such guidelines are available from other CDC data collection activities56.
Your EHDI program could consider the following components7in an analysis plan.
- A profile of the jurisdiction's data needs and priorities;
- A listing of the planned types of analyses and the rationale for each;
- The policy for the review of reports, presentation, and manuscripts;
- The policy for access to data by external researchers, if needed; and
- The authorship policy.
The following sections will explain what a data analysis plan is and the key items to consider during its development. Suggestions on creating the analysis file and possible data analyses to consider will also be examined.
What is a data analysis plan (DAP)?
A DAP is used as a guide for planning and conducting a specific data analysis. It is used to determine the type of data analysis that will be performed on a dataset, what software or program will be used, and who is responsible for the tasks. It also discusses how the results will be used, the timeline for completing the task, and the dissemination plan.
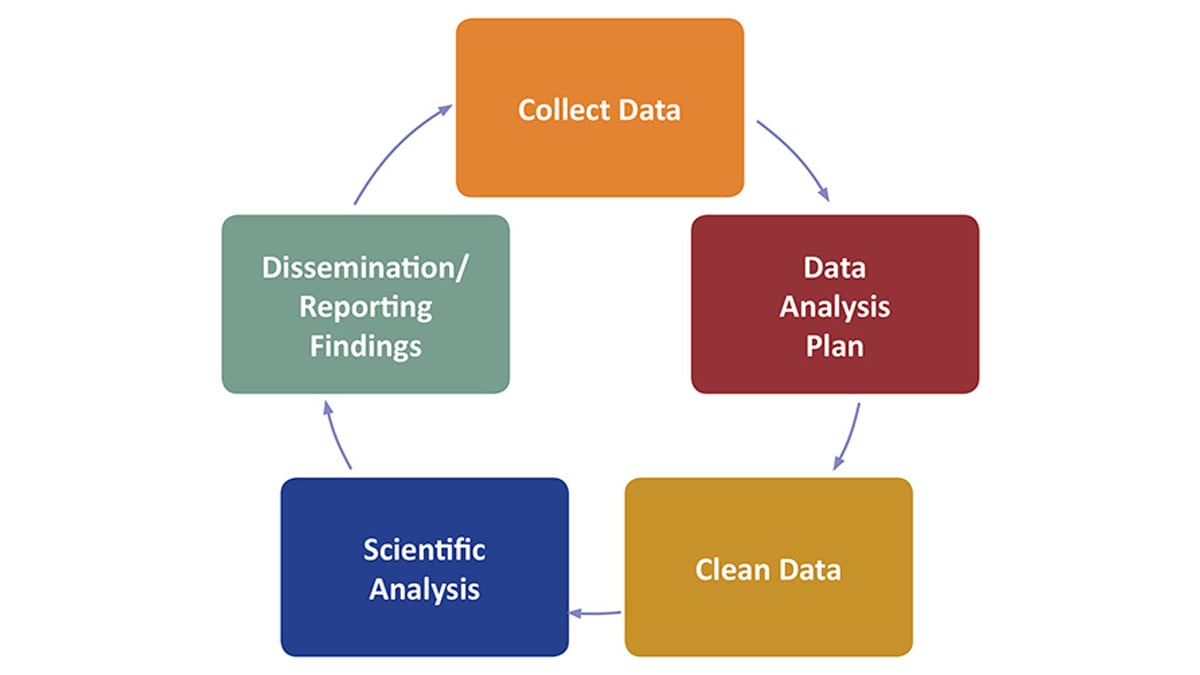
What are some key considerations in developing an overall analysis plan or DAP?
Things to consider include
- Who will be cleaning or reformatting the data for analysis?
- Will data not included in the EHDI-IS be required and, if so, will that be readily available?
- Will data not readily available, such as that from a partner agency, be needed? Will it be possible to get?
- Do any agreements need to be drafted and signed (e.g., a memorandum of agreement)?
- Who will actually conduct the analyses and how much time will they take?
- A timetable for creating data files (including incorporating any data not already in the EHDI-IS), the preliminary analysis and report, and the final analysis and report. The table can also specify who will be completing each task (e.g., IT, data analyst, and writer).
- Does the project require Institutional Review Board (IRB) review for analyses and/or the release of findings?
When completing more in-depth analysis, a DAP may include
- Research question(s) or hypothesis;
- Inclusion/exclusion criteria, including the data's time frame (e.g., 2013 births);
- Variables to be used in the analysis;
- Format of data files and format of the variables;
- Statistical methods and software to be used to analyze the data;
- Table shells for the results;
- Dissemination plans;
- Person(s) responsible for the analysis; and
- A timeline for completion.
Main components of a DAP
A DAP provides a map of the planned analysis and is often developed step-by-step. A DAP is made up of four main components: background, aims/objectives, methods, and planned tables and figures.
Background
It is important to look at what has been done in other studies. The background section provides an overview of the relevant literature and the rationale for the study. The rationale provides justification of the research questions and why the analysis method was chosen.
Aims/objectives
The research questions (aims/objectives) are often clearly defined as testable hypotheses. Hypotheses are the bridge between questions and data. Data may confirm or refute a hypothesis.
Methods
The methods section typically include details on the following
- Data sources;
- Definition of the study population and outline of the inclusion/exclusion criteria;
- Detailed definitions, including categorization of study measures that contain the main exposure variables, outcome variables and other covariates (including confounders and mediators);
- Sub-groups in which you may wish to explore any variation of main effects;
- Details about methods for cleaning or handling missing data (e.g., complete case analysis, coding missing values as separate categories, imputation methods and/or sensitivity analyses);
- Details about sensitivity analyses to be undertaken;
- Planned analyses including the statistical methods used, how hypotheses will be tested, and how potential confounding and bias will be assessed and addressed;
- Statistical methods for planned model building, methods to verify statistical assumptions and alternative methods to be adopted; and
- Specifications of the statistical software used and the version.
Planned tables and figures
Planned tables and figures are also referred to as dummy tables and figures, which are skeletons that provide an outline of how the results will be presented. The dummy tables contain empty cells that will be populated after the data analysis.
Having planned tables and figures
- Brings focus on what you are doing and how you will display your results;
- Provides a useful talking point for discussing the analyses with collaborators;
- Allows for refinement of your research aims; and
- Can be copied directly into the results section with cells populated after analysis.
Choosing the right statistical software
There are a variety of statistical software packages that may be used to answer questions with your EHDI-IS data. A list of statistical software is listed here. 8Data conversion software, such as StatTransfer9, can be used to quickly convert data files from one package to another. A comparison of the most common statistical software is shown in Table 1.
Each statistical package offers its own unique strengths and weaknesses. For example, EpiInfo can be used to provide both aggregate and individual-level descriptive statistics. If you were performing analyses using mixed models, you might choose SAS. However, if you were doing logistic regression, you might choose Stata. If you were doing analysis of variance, you might choose SPSS. The right statistical software depends on what your program needs and budget constraints. This is where an overall analysis plan comes in handy in assessing what analyses will be expected.
When it comes to selecting a specific software for your EHDI program, the right statistical software depends on several factors:
- Who will be using the statistical software?
- What are the costs for software?
- How easy is the software to use?
- Where will people use the statistical software?
- What kind of support do your statistical software users want?
- What questions are you trying to answer and what analysis are you interested in?
Table 1: Comparison of the Most Common Statistical Software Features and Capabilities
Statistical Software | Mac / Windows | Interface* | Learning Curve | Data Manipulation | Statistical Analysis | Graphics | Specialties |
---|---|---|---|---|---|---|---|
Epi Info™ | Both | Menus & Syntax | Gradual | Moderate | Broad Scope Medium | Very Good | Easy data entry and construction for small to mid-sized disease surveillance systems, epidemiologic statistics, maps, and graphs for those with limited technology experience |
The R Project for Statistical Computing (R) | Both | Syntax | Steep | Very Strong | Very Broad Scope High Versatility |
Excellent | Packages for Graphics, Web Scraping, Machine Learning & Predictive Modeling |
Statistical Applications Software (SAS) | Windows | Syntax | Steep | Very Strong | Very Broad Scope High Versatility |
Very Good | Large Datasets, Reporting, Password Encryption & Components for Specific Fields |
SPSS | Both | Menus & Syntax | Gradual | Moderate | Moderate Scope Low Versatility |
Good | Custom Tables, ANOVA & Multivariate Analysis |
Stata | Both | Menus & Syntax | Moderate | Strong | Broad Scope Medium |
Good | Panel Data, Survey Data Analysis & Multiple Imputation |
* The primary interface is bolded when multiple interfaces available.
Who are the users?
The first thing to consider in choosing the right statistical software package is determining who could or will use the software. Will the users be expert statisticians, relative novices, or a mix of both? Will the users be expected to analyze data on a more frequent basis (daily or weekly) or less frequent basis (quarterly or semi-annually)? Is data analysis a core part of their jobs or just one of the many hats that they have to wear? What is the user's relationship with technology – do they like computers or use them because they have to?
Figuring out who will be using the software is key in choosing the appropriate statistical package. This will help you avoid choosing a package that does too much, too little or is completely wrong for your EHDI program.
What are the costs for the statistical software?
The overall analysis plan provides guidance on what the priorities are for the EHDI program and influences what statistical software is most appropriate for use. Budget constraints may limit statistical software choices and licensing fees for statistical software can be expensive. However, R and Epi-Info are free non-proprietary options.
Ease of use–novice or expert
Data analysis is not made easier by the statistical software package. The software's ease of use depends on the user. An expert statistician will know how to set up data correctly and will be comfortable entering statistical equations in a command-line interface. They may feel slowed down by using a menu-based interface. Conversely, a less experienced user may be intimidated or overwhelmed by a statistical software package designed primarily for expert users. Novices may feel more comfortable with a menu-based interface.
To see which statistical software package works best for the majority of your users, compare the interface options that are offered. Is it possible to customize the statistical software package for users who have different skill sets? Does the package offer a streamlined interface for novices as well as the command line for users who prefer it?
The good news is that statistical software packages are easier to learn than they used to be. Most statistical software offer tutorials and documentation on their respective websites or through user forums that should help when questions arise.
Where will the statistical software be used?
After determining who the users are, determine where the analyses will occur. What are the license requirements and how many licenses will you need? What are the costs of the licenses? Check with the software provider if you need to purchase a separate license for each computer or if shared licenses are available. A good software provider will work with you to understand your program's needs and find the most cost-effective solution.
Expected support
If users in your organization will need to contact the software's support team for assistance, it is important to check and see what types of assistance are offered. Will users need help using statistical software to analyze their data? Does your program have expert statisticians who can provide assistance when it is needed or is access to that expertise limited?
Compare the technical support options offered. Will they help with analysis problems or only with installation and IT issues? Is there additional charge for technical support? While some software packages offer free technical support from experts in statistics and IT; others may provide more limited, fee-based customer support or no support at all.
Uses for the software
The right statistical software program will help you answer the questions that you want and complete the analysis that you are interested in. Table 2 shows the different types of statistical analyses that can be performed using R, SAS, SPSS, and STATA. It is possible to integrate two statistical software programs to analyze your data, i.e., SPSS Statistics and R.
Table 2: Summary of Statistical Analysis by Statistical Program
Type of Statistical Analysis | R | SAS | SPSS | STATA |
---|---|---|---|---|
ANCOVA & MANCOVA | Yes | Yes | Yes | Yes |
ANOVA & MANOVA | Yes | Yes | Yes | Yes |
Bayesian Statistics | Yes | Limited | ||
Bootstrap & Jackknife | Yes | Yes | Yes | Yes |
Canonical Correlation Analysis | Yes | Yes | Yes | Yes |
Classification & Regression Trees | Yes | Yes | Limited | |
Cluster Analysis | Yes | Yes | Yes | Yes |
Counting Processes | Yes | Yes | ||
Cross-Validation | Yes | Yes | ||
Deterministic Optimization | Yes | Yes | Limited | |
Discriminant Analysis | Yes | Yes | Yes | Yes |
EM Algorithm | Yes | Yes | ||
Factor & Principal Components Analysis | Yes | Yes | Yes | Yes |
Generalized Least Squares | Yes | Yes | Yes | Yes |
Generalized Linear Models | Yes | Yes | Yes | Yes |
Hidden Markov Models | Yes | |||
Instrumental Variables | Yes | Yes | Yes | Yes |
Lasso | Yes | Yes | Limited | |
Linear Regression | Yes | Yes | Yes | Yes |
Logistic Regression | Yes | Yes | Yes | Yes |
Longitudinal (Panel) Data | Yes | Yes | Limited | Yes |
Markov Chain Monte Carlo | Yes | Yes | ||
Markov Chains | Yes | |||
Missing Data Imputation | Yes | Yes | Yes | Yes |
Mixed Effects Models | Yes | Yes | Yes | Yes |
Monte Carlo, Classic Methods | Yes | Yes | Limited | Yes |
Multivariate Time Series | Yes | Yes | Yes | |
Nonlinear Regression | Yes | Yes | Limited | Limited |
Nonparametric Smoothing Methods | Yes | Yes | Yes | |
Nonparametric Tests | Yes | Yes | Yes | Yes |
Outlier Diagnostics | Yes | Yes | Yes | Yes |
Path Analysis | Yes | Yes | Yes | Yes |
Propensity Score Matching | Yes | Limited | Limited | |
Quality Control | Yes | Yes | Yes | Yes |
Random Forests | Yes | Limited | ||
Reliability Theory | Yes | Yes | Yes | Yes |
Ridge Regression | Yes | Yes | Limited | Limited |
Robust Estimation | Yes | Yes | Yes | |
ROC Curves | Yes | Yes | Yes | Yes |
Signal Processing | Yes | |||
Simultaneous Equations | Yes | Yes | Limited | Yes |
Stochastic Optimization | Yes | Limited | ||
Stochastic Volatility Models, Continuous Case | Yes | Limited | Limited | |
Stochastic Volatility Models, Discrete Case | Yes | Yes | Limited | Yes |
Stratified Samples (Survey Data) | Yes | Yes | Yes | Yes |
Structural Equation Modeling (Latent Factors) | Yes | Yes | Yes | Yes |
Survival Analysis | Yes | Yes | Yes | Yes |
T-test | Yes | Yes | Yes | Yes |
Univariate Time Series | Yes | Yes | Limited | Yes |
Variance Stabilization | Yes |
Creating an analysis file
What is an analysis file?
An analysis file is the data file used by the person conducting the analysis or using the data for research. Generally, the analyst uses an analysis file that is stripped of any identifying information (the 18 elements specified in 45 CFR §164.514 (e)(2) [PDF – 154KB]). The ONLY possible exception of creating a de-identified file is if the analysis is done on a jurisdiction's secure server. According to 45 CFR §164.514 (e)(2) [PDF – 154KB], a limited data set is protected health information that excludes the following direct identifiers of the individual or of relatives, employers or household members: names, postal address, telephone numbers, fax numbers, electronic mail addresses, social security numbers, medical record numbers, health plan beneficiary numbers, account numbers, certificate/license numbers, vehicle identifiers and serial numbers, device identifiers and serial numbers, web universal resource locators, internet protocol address numbers, biometric identifiers including fingerprints and voice, and full face photographic images and any comparable images.
If the analyst is using a laptop, even if it is a "jurisdiction" laptop, all identifying information is normally stripped away for security. Ideally, even the official person-ID numbers used by the EHDI-IS or state data systems is changed to a new random number. This would make the data unidentifiable to anyone other than the person who created the file. Detailed recordkeeping of the random number assignments are maintained by the person who created the data file or the data manager. If a discrepancy occurs in the data, the individual can go back to original record to verify and validate the information.
The full guidance regarding methods for de-identification of protected health information in accordance to the HIPAA Privacy Rule can be found in section titled De-Identification Standard for Protected Health Information of Chapter 5. De-identification refers to the process by which identifiers are removed from EDHI-IS data. Not only does this mitigate risks to the individuals, it also supports the secondary use of data for additional use, i.e. follow-up and early intervention enrollment. A summary of the HIPAA Privacy Rule can be found here [PDF – 372KB]. 10More information on protected health information can be found in Chapter 5.
Consider having procedures in place for data sharing. Will the EHDI-IS aggregate data be publicly available? For researchers who want access to individual EHDI-IS data, what procedures will be in place to gain access? For more specifics on the importance of having relevant and clear policies in place for sharing and protecting EHDI-IS information, refer to Chapter 5.
Cleaning the data file
The data cleaning checklist includes
- Validate the accuracy of the data;
- Address missing data;
- Remove univariate and multivariate outliers; and
- Assess for normality.
Accuracy of the data
Accuracy is the degree to which data correctly describes the "real world" object or event being described. It is one of the main components of data quality. Possible questions include: Was the data recorded correctly? Check the numeric values, date values, and spelling of the data. Check for duplicates. Does the number of the births reported in the EHDI-IS match the number of births in the state's Vital Records System? What is the status of the baby? Has the baby undergone hearing screening, diagnostic evaluation or been enrolled in early intervention? Has the status of the baby been correctly entered into the EHDI-IS? For more information on data quality, refer to the EHDI-IS Functional Standards.
Missing data
Missing data is the absence of an observation for a variable. The most important thing in dealing with missing data is to determine whether its absence is random or if there is some pattern (reason) that the data is missing. It may be necessary to label each missing value with the reason it is considered missing in order to guarantee accurate bases for analysis. Remedies to missing data include dropping the observations with missing data, or completing a mean substitution or a multiple imputation.
Removal of outliers
Outliers are unusually large or small values that are dramatically separated from the rest of the data. They might be "out-of-range" or physically impossible values that resulted from an entry or processing error. Consider removing invalid, impossible, or extreme values from the dataset. Outliers might also be marked for exclusion for the purpose of certain analyses.
Reasons for an outlier include
- A mistake in data entry;
- Missing values that were not specified and are being read as case entries;
- The outlier is not part of the population you want to sample; or
- The outlier is part of the population that you want to study but is an extreme case.
Normality
Normality refers to the distribution of the values (e.g., the shape of a normal bell curve). The distribution is a summary of the frequency of individual values or ranges of values for a variable. A check for normality is needed for many statistical procedures, especially parametric tests, because the validity of these tests are based on the assumption of normality (i.e., populations from which the sample population is taken is normally distributed). It is critical to assess normality when performing statistical tests (e.g., means, medians, or testing differences between groups). However, if the distribution is not normal, you can do data transformations or run nonparametric tests. For example, you may want to compare the means between two distinct or independent groups (e.g., comparing the mean systolic blood pressure at baseline for patients assigned to the placebo and those assigned to the treatment group). In such as case, you can run a two-sample t-test for a parametric procedure and a Wilcoxon rank-sum test for a non-parametric procedure.
Choosing the format of the analysis file
The file format will depend on the type of analysis being conducted and the specific software being used. Consider confirming the file formats with the person doing the analysis before generating the analysis files. Ideally, your EHDI-IS will be able to easily generate files in multiple formats. If it cannot, you may want to consider incorporating this feature or using data conversion software, such as StatTransfer. This is a software that allows data to quickly move between worksheets, databases, and statistical packages.
Longitudinal versus repeated measures format
If multiple assessments are included for the same variable (e.g., data on multiple screenings for the same child), how do these multiple assessments appear in a data file?
Longitudinal design
- Each row represents one person and the multiple assessments appear as multiple variables (e.g., screen1, screen2, screen3, etc.).
- The number of these variables will equal the maximum number of assessments for that variable (e.g., if the maximum number of screens was 8, there would be variables screen1 up to screen8).
- The number of these variables will equal the maximum number of assessments for that variable (e.g., if the maximum number of screens was 8, there would be variables screen1 up to screen8).
Repeated measures design
- Each row represents one assessment, so the same child will appear in multiple rows.
- Each row includes a single variable (screen) for the assessment and an additional variable indicating which assessment it was for that child (e.g., Assessment 5).
- The number of rows for a child will be equal to the number of assessments the child received.
Multilevel format
A multilevel format is similar to the "repeated measures" format, where variables that correspond to each level appear in their own data file.
What is nested data?
Nested data occurs when participants and/or assessments are contained or organized into larger groups of similar kinds or entities that do not overlap. For nested data, multiple data files are created for each level of nesting. For example:
- Births can be grouped by hospital.
- Each child is born in only one hospital (potentially confounded by transfers).
- Births are "nested" within hospitals.
- Each child is born in only one hospital (potentially confounded by transfers).
- Two data files are created.
- Hospital File: Contains hospital variables with the number of records equal to the number of hospitals.
- Child File: Contains child variables with the number of records equal to the number of children. The child file contains a hospital ID number so that children can be linked to their hospital of birth.
- Hospital File: Contains hospital variables with the number of records equal to the number of hospitals.
Multiple assessments (e.g., multiple screenings) for the same child are grouped by child.
- Each screening result applies to only one child.
- Screenings are "nested" within children.
- Two data files are created.
- Child File: Contains child variables with the number of records equal to the number of children.
- Screening File: Contains screen-specific variables with the number of records equal to the total number of screenings conducted. The screening file also contains a Child ID number so that screenings are linked to the specific child who was screened.
- Child File: Contains child variables with the number of records equal to the number of children.
"Level" of the Analysis. Each of these groupings is considered a "level" of the analysis.
- Births nested within hospitals
- Child level: Variables are unique to each child, such as the sex, race, insurance status, NICU status, etc.
- Hospital level: Variables are unique to each hospital, such as its size, number of births, type of screening performed, etc.
- Child level: Variables are unique to each child, such as the sex, race, insurance status, NICU status, etc.
- Multiple screenings nested within children
- Assessment level: Variables are unique to each screening, such as the result, the time since birth, etc.
- Child level: Variables are unique to each child, such as the sex, race, and insurance status of the child.
Recognizing nesting is important when the research question asks "How does something at one level influence something at a different level?" For example:
- What hospital characteristics are related to higher risk of loss to follow-up?
- What child characteristics are related to higher numbers of screenings?
Exporting data in an appropriate, user-friendly format
From an IT design perspective, information in a database is often stored or coded in ways that are actually very inconvenient for statistical analysis. Ideally, the EHDI-IT staff and the person conducting the analyses will sit down before creating the analysis file and determine the characteristics of individual data fields and how categorical data will be coded.
If you are considering creating some generic data export capacity for your EHDI-IS, a meeting with a statistician early in the process is wise to have her/him suggest how those data should be formatted for export. This may involve a variety of preliminary queries and data modifications may occur as part of the data export process.
Consider familiarizing everyone involved in data analysis with your EHDI program and the jurisdiction's data collection protocol. The analytic file could also include a codebook that identifies how all the variables are coded. Any limitations regarding the data could be explained and taken into account during the analysis as needed. For example, limitations may include the inability to generalize the data to other states.
Using EHDI data to answer your questions
What questions can EHDI data answer?
Possible questions that your EHDI-IS program can address include program operations, case management/processing, and descriptive information on babies captured in the EHDI-IS. Below are some examples for each area.
- Program operations. This might include analyses examining data quality and completeness, as well as efficiency and use of resources. The latter might include things such as what are the diminishing returns of repeated contact attempts with families (i.e., with each successive attempt, you get fewer people engaged. At some point, it's not worth continuing to try–what is that threshold number of attempts?).
- Case management/processing. Questions can be answered, for example, about the timeliness of services throughout the follow-up period. Are infants being diagnosed by 3 months of age and starting intervention before 6 months of age? Are there sub-populations or regions being underserved? Is there a differential loss to follow-up/loss to documentation (LFU/LTD) for some groups?
- Descriptive information on babies captured in the EHDI-IS. Additional information that may be captured in the EHDI-IS include:
- Family history;
- Genetic disorders;
- Problems with the structure of the inner ear;
- Low birth weight;
- Birth defects;
- Racial and ethnic distributions;
- Socioeconomic status;
- Insurance status;
- Other comorbidities
- Family history;
Standardizing EHDI data reporting
Each year, the CDC EHDI program collects aggregate information from jurisdictions for the Hearing Screening and Follow-up Survey (HSFS). The HSFS is a voluntary survey that gathers non-estimated data related to the receipt of hearing screening, diagnostic testing and EI enrollment for all occurrent births within a jurisdiction in a given year. The HSFS not only allows CDC to monitor progress toward 1-3-6 benchmarks, but also monitor progress in other needed areas, e.g., number of infants who did not receive or were not documented as receiving recommended follow-up services (infants LFU/LTD). Lack of standardization in reporting screening and diagnostic data and inconsistent definitions have contributed to some infants becoming LFU/LTD. 11It is difficult to properly assess progress toward the EHDI 1-3-6 benchmarks when the local data is incomplete or inconsistent.
To ensure that D/HH infants are receiving timely services, it is crucial that the EHDI data reported from hospitals, audiologists, and other providers is complete, consistent, and accurate. Several efforts have been made to address the lack of standardization including updates to the HSFS (i.e., defining LFU/LTD, in progress, confirmed hearing loss), the creation of the EHDI-IS Function Standards, and support of national standardization initiatives to improve interoperability (e-measures and quality measures). For more information on standardizing and reporting EHDI data and progress towards it, go here.
EHDI data reporting among providers and jurisdictional programs
Under the HIPAA Privacy Rule, covered entities can disclose protected health information without authorization to public health authorities who are legally authorized to receive such reports for the purpose of preventing or controlling disease, injury or disability. This includes the reporting of a disease or injury, reporting vital events, such as births or deaths, and conducting public health surveillance, investigations, or interventions. Under §164.502(a)12of the Privacy Rule [PDF – 154KB], signed consent is not needed for public health authorities to share newborn hearing screening information. A covered entity is permitted to use or disclose protected health information to the individual or for the treatment, payment, or health care operations, as permitted by and in compliance with § 164.506. Hence, personal information can be shared for public health purposes (e.g., surveillance of newborn hearing screening outcomes) without signed consent. Because EHDI is considered a public health authority, EHDI programs can obtain personally identifiable screening information without signed consent from hospitals and health care providers who perform the screening. Hospitals may also provide hearing screening information to primary health providers and diagnosticians without signed consent to facilitate treatment. For more information on uses and disclosures of data to carry out treatment, payment, or health care operations (§164.506), go here.
Data dissemination
Disseminating EHDI data is an important public health function. Sharing individual-level data among providers helps better serve patients by improving documentation and follow-up practices. Reporting aggregate-level data helps with program improvement and advocacy. Reporting data to other stakeholders (e.g., hospital administrators) may motivate them to spend more time and effort collecting and reporting the data to your EHDI program. If the quality of the data is improved, jurisdictions will be able to make more informed decisions about their needs including whether policy changes are necessary. More detailed information regarding the impact of federal regulations on information sharing among providers and parents (i.e., HIPAA, Family Educational Rights and Privacy Act (FERPA), and Part C) is provided in Chapter 5.
How will you disseminate the data results, and with whom?
Consider having a dissemination plan in place. Methods for disseminating EHDI-IS data include:
- Presentations to the advisory board;
- Presentations and reports to stakeholders (e.g., legislators, Part C, partners);
- Reports to providers (e.g., hospitals, audiologists, EI programs, etc.);
- Newsletters;
- Posting on the EHDI website;
- Journal publications;
- Webinars;
- Social media.
Before presenting the results, it is advisable to follow any established jurisdictional and agency rules regarding dissemination.
How does the EHDI program disseminate data to hospitals, parents and providers?
Reporting aggregate data is beneficial for all parties involved in an EHDI program. This
- Supports the provision of services;
- Promotes awareness;
- Improves collaboration and coordination among stakeholders;
- Allows for opportunities to identify and address gaps; and
- Improves practices.
EHDI programs may choose to report aggregated EHDI data or tabulated results with hospitals and health care providers to monitor reporting and follow-up for those who do not pass hearing screening. Consider developing standard hospital procedures for sharing information with the child's healthcare provider, guardians/parents, EHDI programs, and for coordinating referrals among EHDI programs and Part C. Sharing reports of their progress of achieving the EHDI's 1-3-6 benchmarks allows programs to assess their success. Specific information regarding recommended practices for sharing screening, diagnostic, and EI data among providers is covered in more detail in Chapter 5.
Various methods can be used to disseminate data to hospitals and providers, such as
- Hospital report cards;
- Audiology report cards;
- Email;
- Letters; or
- Presentations during site visits.
Regardless of the method chosen, your program could include statistics for the hospital or provider in terms of their performance:
- In providing services and reporting results to their jurisdictional EHDI program;
- On how they compare with the rest of the hospitals and/or providers within the jurisdiction; and
- On whether the entity has improved, using statistics from the previous year for comparison.
Disseminating data with other stakeholders may be a little different. How data is reported depends on the purpose of why it is being shared. Most EHDI programs provide tabulated data results with stakeholders through their EHDI website, presentations at conferences, or through informational products (i.e., brochure, pamphlet). The stakeholders may be involved in developing the product to ensure that the end product meets all expectations and needs. Data to include may primarily consist of national statistics, similar to what is reported in the summaries found here.13
Providing EHDI data to parents may be done via newsletter, listserv, email, website, mail or in-person. Parents/guardians can be informed of their child's screening results immediately after the hearing screening occurs. Additional data to share with parents include statistics of LFU/LTD for diagnosis and LFU/LTD for EI, screening rates, diagnostic rates, and EI enrollment rates. This may help promote awareness among the parents and encourage them to be proactive in meeting the 1-3-6 benchmarks for their child.
How to build data reports: the right data, to the right people, In the right format, and at the right time
The right data
Chapters 2, 3, and 4 have focused on developing your EHDI-IS system and processes in order to obtain quality data that describe the screening, diagnostic assessment results, and early intervention outcomes for all infants within your jurisdiction. It is important to consider as well the way you share this data with other EHDI stakeholders in order to maximize the impact and increase the likelihood that they will understand and act on your recommendations. Decide on the core message for your audience and what you would like them to do after they have seen the data. Then select data that contribute to that objective.
To the right people
It will be important to consider the recipient of your data report and what will be most useful. Potential audience members include:
- Parents
- Parents will often be most interested in the individual results for their child. They may be interested in findings and test results along with recommendations for future steps to take in the care of their child. Consider that parents may hear the results and recommendations from their infant's provider, but sometimes benefit from "hearing" it a second time from the EHDI program.
- Parents will often be most interested in the individual results for their child. They may be interested in findings and test results along with recommendations for future steps to take in the care of their child. Consider that parents may hear the results and recommendations from their infant's provider, but sometimes benefit from "hearing" it a second time from the EHDI program.
- Providers
- Providers will most often be most interested in
- 1. Individual results for specific patients at risk for, or diagnosed with hearing loss
- 2. Recommendations and "next steps" for treatment and care of the patients in their caseload
- They may also want to see aggregated results and trends that describe the performance of their clinic or institution.
- Providers will most often be most interested in
- Program and policy makers
- Program staff and agency leaders, as well as other policy makers, are often most interested in data that reflect the status of the EHDI program in their jurisdictions.
- They may want to know the performance of specific areas of the EHDI program, such as whether the 1-3-6 benchmarks and timelines are being met for every child born in the jurisdiction.
- They may also seek out data from the EHDI program that has been merged with other public health data. Vital statistics data, demographic data, and socioeconomic data can provide additional understanding and context that help leaders make programmatic decisions.
- Program staff and agency leaders, as well as other policy makers, are often most interested in data that reflect the status of the EHDI program in their jurisdictions.
In the right format
It is worth spending time to make the report or presentation easy to use and understand. Doing so will allow your audience to spend their time and energy on the content, rather than figuring out the structure of the report.
Keep in mind the way your audience will be getting the information. Content will need to be organized and designed differently for a fact sheet, a website or a PowerPoint presentation.
Break content into manageable, logical "chunks" for easier access and label these sections clearly.
When building a report or presentation, consider using the CDC's Clear Communication Index. The CDC Clear Communication Index is a research-based tool to help you develop public communication materials that audiences will readily understand.
In selecting the types of charts and graphs to use, consider first the types that are familiar to your audience and then choose the particular chart that fits your data. Suggestions for matching charts to different data types can be found at: http://www.cancer.gov/publications/health-communication/making-data-talk.pdf [PDF – 2.03MB].
Consider efforts to ensure your message cannot be misinterpreted. Avoid noted common mistakes below that can confuse your audience or distort your message.
- Keep your visuals clean and free from clutter. Eliminate anything that distracts from the point the data is making.
- Avoid using 3-D or special effects, even if these are available in your report-making software.
- Include any context that is important to understanding the findings, such as historical results. A sharp short-term drop may look dramatic in isolation but may be much less striking when compared to long-term experience.
- Start the Y axis at zero, or make it very clear that you have done otherwise.
- Avoid using varying sizes of a picture to indicate change over time. Readers find it difficult to judge the relative areas of objects. They may also be confused about whether the height of the object or its volume represents the relative change and may easily misinterpret the findings.
At the right time
Timely data reports are essential to effective decision-making in every aspect of your EHDI program. The availability of timely data and reports will help parents and providers make better choices regarding the care of their children and patients. Timely data will improve discussion about the effectiveness of your program and help program staff and policy makers determine best levels of support. Consider how you might modify your EHDI-IS in order to report the relevant data as promptly as possible.
- EHDI Functional Standards
- Title IV—Prevention of Chronic Disease and Improving Public Health [PDF – 241KB]
- Data Collection Standards for Race, Ethnicity, Sex, Primary Language, and Disability Status
- CDC's Creating and Analysis Plan – Participant Workbook [PDF – 532 KB]
- Are PRAMS data available to outside researchers?
- CDC's Research Data Center (RDC)
- Developing a Quantitative Data Analysis Plan for Observational Studies
- List of Statistical Software
- Stat/Transfer
- Summary of the HIPAA Privacy Rule [PDF – 373 KB]
- Alam S, Satterfield A, Mason CA, and Deng X (2016). Progress in Standardization of Reporting and Analysis of Data from Early Hearing Detection and Intervention (EHDI) Programs. Journal of Early Hearing Detection and Intervention 1(2): 2-7. PMCID: PMC5102258
- 164.502 Uses and disclosures of protected health information: general rules [PDF – 153 KB]
- Annual Data Early Hearing Detection and Intervention (EHDI) Program